图书介绍
非线性时间序列分析 第2版 英文2025|PDF|Epub|mobi|kindle电子书版本百度云盘下载
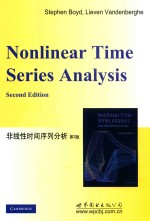
- (德)坎兹著 著
- 出版社: 北京:世界图书北京出版公司
- ISBN:9787510087721
- 出版时间:2015
- 标注页数:369页
- 文件大小:85MB
- 文件页数:388页
- 主题词:非线性-时间序列分析-英文
PDF下载
下载说明
非线性时间序列分析 第2版 英文PDF格式电子书版下载
下载的文件为RAR压缩包。需要使用解压软件进行解压得到PDF格式图书。建议使用BT下载工具Free Download Manager进行下载,简称FDM(免费,没有广告,支持多平台)。本站资源全部打包为BT种子。所以需要使用专业的BT下载软件进行下载。如BitComet qBittorrent uTorrent等BT下载工具。迅雷目前由于本站不是热门资源。不推荐使用!后期资源热门了。安装了迅雷也可以迅雷进行下载!
(文件页数 要大于 标注页数,上中下等多册电子书除外)
注意:本站所有压缩包均有解压码: 点击下载压缩包解压工具
图书目录
Ⅰ Basic topics1
1 Introduction:why nonlinear methods?3
2 Linear tools and general considerations13
2.1 Stationarity and sampling13
2.2 Testing for stationarity15
2.3 Linear correlations and the power spectrum18
2.3.1 Stationarity and the low-frequency component in the power spectrum23
2.4 Linear filters24
2.5 Linear predictions27
3 Phase space methods30
3.1 Determinism:uniqueness in phase space30
3.2 Delay reconstruction35
3.3 Finding a good embedding36
3.3.1 False neighbours37
3.3.2 The time lag39
3.4 Visual inspection of data39
3.5 Poincaré surface of section41
3.6 Recurrence plots43
4 Determinism and predictability48
4.1 Sources of predictability48
4.2 Simple nonlinear prediction algorithm50
4.3 Verification of successful prediction53
4.4 Cross-prediction errors:probing stationarity56
4.5 Simple nonlinear noise reduction58
5 Instability:Lyapunov exponents65
5.1 Sensitive dependence on initial conditions65
5.2 Exponential divergence66
5.3 Measuring the maximal exponent from data69
6 Self-similarity:dimensions75
6.1 Attractor geometry and fractals75
6.2 Correlation dimension77
6.3 Correlation sum from a time series78
6.4 Interpretation and pitfalls82
6.5 Temporal correlations,non-stationarity,and space time separation plots87
6.6 Practical considerations91
6.7 A useful application:determination of the noise level using the correlation integral92
6.8 Multi-scale or self-similar signals95
6.8.1 Scaling laws96
6.8.2 Detrended fluctuation analysis100
7 Using nonlinear methods when determinism is weak105
7.1 Testing for nonlinearity with surrogate data107
7.1.1 The null hypothesis109
7.1.2 How to make surrogate data sets110
7.1.3 Which statistics to use113
7.1.4 What can go wrong115
7.1.5 What we have learned117
7.2 Nonlinear statistics for system discrimination118
7.3 Extracting qualitative information from a time series121
8 Selected nonlinear phenomena126
8.1 Robustness and limit cycles126
8.2 Coexistence of attractors128
8.3 Transients128
8.4 Intermittency129
8.5 Structural stability133
8.6 Bifurcations135
8.7 Quasi-periodicity139
Ⅱ Advanced topics141
9 Advanced embedding methods143
9.1 Embedding theorems143
9.1.1 Whitney's embedding theorem144
9.1.2 Takens's delay embedding theorem146
9.2 The time lag148
9.3 Filtered delay embeddings152
9.3.1 Derivative coordinates152
9.3.2 Principal component analysis154
9.4 Fluctuating time intervals158
9.5 Multichannel measurements159
9.5.1 Equivalent variables at different positions160
9.5.2 Variables with different physical meanings161
9.5.3 Distributed systems161
9.6 Embedding of interspike intervals162
9.7 High dimensional chaos and the limitations of the time delay embedding165
9.8 Embedding for systems with time delayed feedback171
10 Chaotic data and noise174
10.1 Measurement noise and dynamical noise174
10.2 Effects of noise175
10.3 Nonlinear noise reduction178
10.3.1 Noise reduction by gradient descent179
10.3.2 Local projective noise reduction180
10.3.3 Implementation of locally projective noise reduction183
10.3.4 How much noise is taken out?186
10.3.5 Consistency tests191
10.4 An application:foetal ECG extraction193
11 More about invariant quantities197
11.1 Ergodicity and strange attractors197
11.2 Lyapunov exponents Ⅱ199
11.2.1 The spectrum of Lyapunov exponents and invariant manifolds200
11.2.2 Flows versus maps202
11.2.3 Tangent space method203
11.2.4 Spurious exponents205
11.2.5 Almost two dimensional flows211
11.3 Dimensions Ⅱ212
11.3.1 Generalised dimensions,multi-fractals213
11.3.2 Information dimension from a time series215
11.4 Entropies217
11.4.1 Chaos and the flow of information217
11.4.2 Entropies of a static distribution218
11.4.3 The Kolmogorov-Sinai entropy220
11.4.4 The ∈-entropy per unit time222
11.4.5 Entropies from time series data226
11.5 How things are related229
11.5.1 Pesin's identity229
11.5.2 Kaplan-Yorke conjecture231
12 Modelling and forecasting234
12.1 Linear stochastic models and filters236
12.1.1 Linear filters237
12.1.2 Nonlinear filters239
12.2 Deterministic dynamics240
12.3 Local methods in phase space241
12.3.1 Almost model free methods241
12.3.2 Local linear fits242
12.4 Global nonlinear models244
12.4.1 Polynomials244
12.4.2 Radial basis functions245
12.4.3 Neural networks246
12.4.4 What to do in practice248
12.5 Improved cost functions249
12.5.1 Overfitting and model costs249
12.5.2 The errors-in-variables problem251
12.5.3 Modelling versus prediction253
12.6 Model verification253
12.7 Nonlinear stochastic processes from data256
12.7.1 Fokker—Planck equations from data257
12.7.2 Markov chains in embedding space259
12.7.3 No embedding theorem for Markov chains260
12.7.4 Predictions for Markov chain data261
12.7.5 Modelling Markov chain data262
12.7.6 Choosing embedding parameters for Markov chains263
12.7.7 Application:prediction of surface wind velocities264
12.8 Predicting prediction errors267
12.8.1 Predictability map267
12.8.2 Individual error prediction268
12.9 Multi-step predictions versus iterated one-step predictions271
13 Non-stationary signals275
13.1 Detecting non-stationarity276
13.1.1 Making non-stationary data stationary279
13.2 Over-embedding280
13.2.1 Deterministic systems with parameter drift280
13.2.2 Markov chain with parameter drift281
13.2.3 Data analysis in over-embedding spaces283
13.2.4 Application:noise reduction for human voice286
13.3 Parameter spaces from data288
14 Coupling and synchronisation of nonlinear systems292
14.1 Measures for interdependence292
14.2 Transfer entropy297
14.3 Synchronisation299
15 Chaos control304
15.1 Unstable periodic orbits and their invariant manifolds306
15.1.1 Locating periodic orbits306
15.1.2 Stable/unstable manifolds from data312
15.2 OGY-control and derivates313
15.3 Variants of OGY-control316
15.4 Delayed feedback317
15.5 Tracking318
15.6 Related aspects319
A Using the TISEAN programs321
A.1 Information relevant to most of the routines322
A.1.1 Efficient neighbour searching322
A.1.2 Re-occurring command options325
A.2 Second-order statistics and linear models326
A.3 Phase space tools327
A.4 Prediction and modelling329
A.4.1 Locally constant predictor329
A.4.2 Locally linear prediction329
A.4.3 Global nonlinear models330
A.5 Lyapunov exponents331
A.6 Dimensions and entropies331
A.6.1 The correlation sum331
A.6.2 Information dimension,fixed mass algorithm332
A.6.3 Entropies333
A.7 Surrogate data and test statistics334
A.8 Noise reduction335
A.9 Finding unstable periodic orbits336
A.10 Multivariate data336
B Description of the experimental data sets338
B.1 Lorenz-like chaos in an NH3 laser338
B.2 Chaos in a periodically modulated NMR laser340
B.3 Vibrating string342
B.4 Taylor-Couette flow342
B.5 Multichannel physiological data343
B.6 Heart rate during atrial fibrillation343
B.7 Human electrocardiogram(ECG)344
B.8 Phonation data345
B.9 Postural control data345
B.10 Autonomous CO2 laser with feedback345
B.11 Nonlinear electric resonance circuit346
B.12 Frequency doubling solid state laser348
B.13 Surface wind velocities349
References350
Index365
热门推荐
- 1066597.html
- 1986034.html
- 2841530.html
- 2989742.html
- 1809248.html
- 673867.html
- 3616852.html
- 1012334.html
- 699189.html
- 1176711.html
- http://www.ickdjs.cc/book_2563422.html
- http://www.ickdjs.cc/book_3701303.html
- http://www.ickdjs.cc/book_2717125.html
- http://www.ickdjs.cc/book_260230.html
- http://www.ickdjs.cc/book_969650.html
- http://www.ickdjs.cc/book_3485860.html
- http://www.ickdjs.cc/book_868944.html
- http://www.ickdjs.cc/book_2827437.html
- http://www.ickdjs.cc/book_646216.html
- http://www.ickdjs.cc/book_2998623.html